OUTPUTS
W RK PACKAGE 5

BenthicNet
Project summary: The manual labelling and classification of benthic imagery is a laborious and subjective process that currently acts as a bottleneck in the benthic habitat mapping workflow. As part of BEcoME Work Package 5, a deep convolutional neural network is being developed to assist in the automated labelling of benthic habitat imagery. Achieving a generalizable model requires a large amount of training data in the form of labelled benthic habitat images. We are compiling a large dataset from existing labelled imagery through collaboration with multiple partners to meet this requirement. At the end of the project, the dataset, model, and outputs will be shared openly.
Data description and approach: The project and dataset are sub-divided into two main components. First, A deep convolutional neural network will be trained on a large volume of unlabeled benthic habitat imagery in order to learn an embedding space to use for transfer learning and for clustering images of similar habitat. The final structure of this dataset will be a collection of seabed images, on the order of 1x10^6, and a single .csv containing the image names and geographic locations/weights. Second, the pre-trained model from the first stage will be transferred to supervised classification tasks. The capacity of the model for transfer learning will be evaluated for both highly specific classification tasks using bespoke classification schemes, and more general tasks using established/transferable schemes (e.g., CATAMI). The data structure for this phase will be sub-collections of seabed images from the first (unsupervised) phase of the project, with .csv files describing the image name, geographic location/weight, and benthic habitat label. Dataset sub-collections will correspond to specific study sites, applications, and thematic levels of classification in order to evaluate the capacity of the model for various classification tasks that are commonly encountered in the benthic habitat mapping literature.
Project status: Image data collection and formatting.
Current data statistics: Image data are currently being compiled. The current dataset characteristics are listed below.
Number of images: 1,534,883
Number of labeled images: 123,103
Number of unique sites (e.g., transects, drifts): >15,786
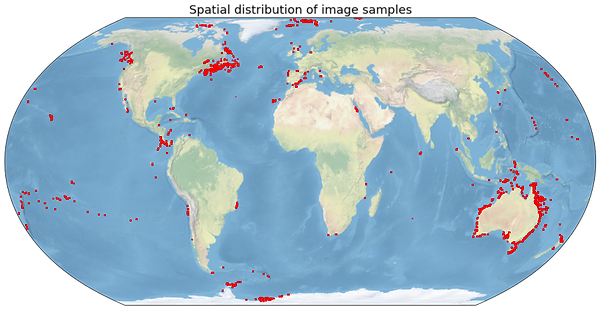